Facial Recognition
AI Training Data For Facial Recognition
Optimize your facial recognition models for accuracy with the best quality image data
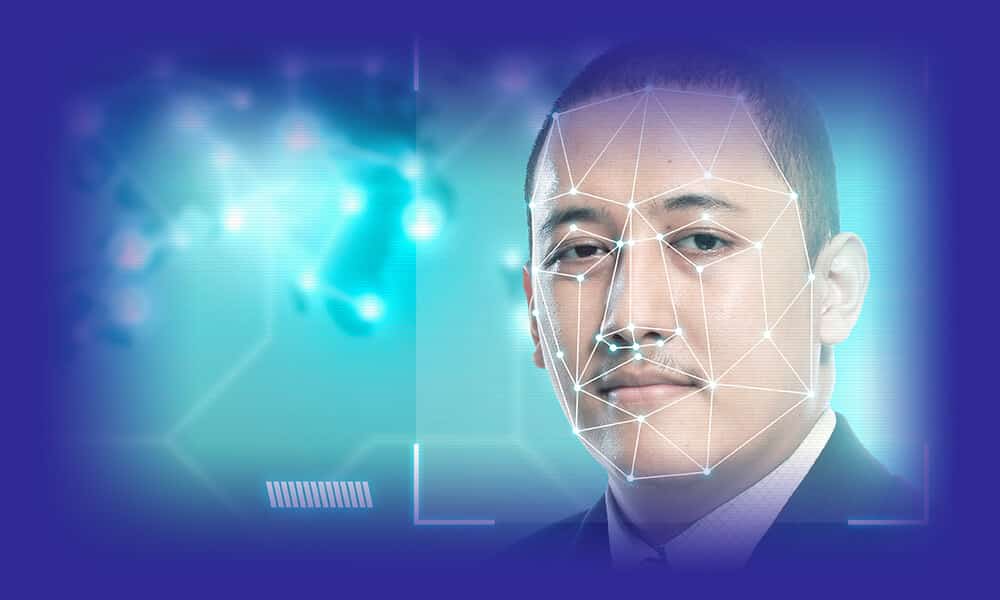
Today, we are at the dawn of the next-generation mechanism, where our faces are our passcodes. Through the recognition of unique facial features, machines can detect if the person trying to access a device is authorized, match CCTV footage with actual images to track felons and defaulters, reduce crime in retail stores, and more. In simple words, this is the technology that scans an individual’s face to authorize access or execute a set of actions it is designed to perform. At the backend, tons of algorithms and modules work at breakneck speeds to execute calculations and match facial features (as shapes and polygons) to accomplish crucial tasks.
The anatomy of an accurate facial recognition model
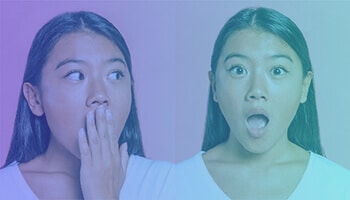
Facial features and perspective
A person’s face looks different from each angle, profile, and perspective. A machine should be able to accurately tell if it is the same person regardless of whether the individual stares at the device regardless from a front-neutral perspective or right-below perspective.
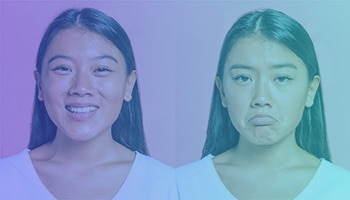
Multitude of facial expressions
A model must precisely tell if a person is smiling, frowning, crying, or staring by looking at them or their images. It should be able to understand that eyes could look the same when a person is either surprised or scared and then detect the precise expression error-free.
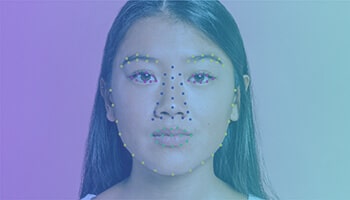
Annotate unique facial identifiers
Visible differentiators like moles, scars, fire burns, and more are differentiators that are unique for individuals & should be considered by AI modules to train and process faces better. Models should be able to detect them and attribute them as facial features and not just skip them.
Facial Recognition Services from Shaip
Whether you need face image data collection (consisting of different facial features, perspectives, expressions or emotions), or face image data annotation services (for tagging visible differentiator, facial expressions with appropriate metadata i.e. smiling, frowning, etc.,) our contributors from across the globe can meet your training data needs fast and at scale.
Face Image Collection
For your AI system to accurately deliver results, it has to be trained with thousands of human facial datasets. The more the volume of image data, the better. That’s why our network can help you source millions of datasets, so your facial recognition system is trained with the most appropriate, relevant, and contextual data. We also understand that your geography, market segment, and demographics could be very specific. To cater to all your needs, we provide face image data across diverse ethnicities, age groups, races, and more. We deploy stringent guidelines on how face images should be uploaded to our system in terms of resolutions, file formats, illumination, poses, and more. This gives us a uniform range of datasets that is not only easy to compile but train as well.
Face Image Annotation
When you acquire quality face images, you’ve completed only 50% of the task. Your facial recognition systems would still give you pointless results (or no results at all) when you feed acquired image datasets into them. To initiate the training process, you need to get your face image annotated. There are several facial recognition data points that have to be marked, gestures that have to be labelled, emotions and expressions that have to be annotated and more. At Shaip, we do all this with precision through our facial landmark recognition techniques. All intricate details and aspects of facial recognition are annotated for accuracy by our own in-house veterans, who have been into the AI spectrum for years.
Shaip Can
Source facial
images
Train resources to label image data
Review data for accuracy & quality
Submit data files in agreed format
Our team of experts can collect and annotate facial images on our proprietary image annotation platform, however, the same annotators after a brief training can also annotate facial images on your in-house image annotation platform. Within a short span, they will be able to annotate thousands of facial images based on stringent specifications and with the desired quality.TE
Facial Recognition Use Cases
Regardless of your idea or market segment, you would need abundant volumes of data that need to be annotated for trainability. So, our solutions will perfectly meet your needs and help speed up your time to market. To get a quick idea of some of the use cases you could reach out to us, here’s a list.
- To implement facial recognition systems in portable devices, IoT ecosystems, and make way for advanced security and encryption.
- For geographical surveillance and security purposes to monitor high-profile neighborhoods, sensitive regions of diplomats, and more.
- To incorporate keyless access to your automobiles or your connected cars.
- To run targeted ad campaigns for your products or services.
- To make healthcare more accessible & turn EHRs interoperable, by granting access through facial features during emergencies & surgeries.
- To offer personalized hospitality services to guests by remembering & profiling their interests, likes/dislikes, room & food preferences etc.
Facial Recognition Datasets / Face Detection Dataset
Face landmark dataset
12k images with variations around head pose, ethnicity, gender, background, angle of capture, age, etc. with 68 landmark points
- Use Case: Facial Recognition
- Format: Images
- Volume: 12,000+
- Annotation: Landmark Annotation
Biometric Dataset
22k facial video dataset from multiple countries with multiple poses for facial recognition models
- Use Case: Facial Recognition
- Format: Video
- Volume: 22,000+
- Annotation: No
Group of People Image Dataset
2.5k+ images from 3,000+ people. Dataset contains images of group of 2-6 people from multiple geographies
- Use Case: Image Recognition Model
- Format: Images
- Volume: 2,500+
- Annotation: No
Biometric Masked Videos Dataset
20k videos of faces with masks for building/training Spoof Detection AI model
- Use Case: Spoof Detection AI model
- Format: Video
- Volume: 20,000+
- Annotation: No
Verticals
Offering facial recognition services to multiple industries
Facial recognition is the current rage across segments, where unique use cases are being tested and rolled out for implementations. From tracking child traffickers and deploying bio ID in organization premises to studying anomalies that could go undetected to the normal eye, facial recognition is helping businesses & industries in a myriad of ways.
Automotive
Healthcare
Retail
Hospitality
Marketing eCommerce
Security & Defense
Our Capability
People
Dedicated and trained teams:
- 30,000+ collaborators for Data Collection, Labeling & QA
- Credentialed Project Management Team
- Experienced Product Development Team
- Talent Pool Sourcing & Onboarding Team
Process
Highest process efficiency is assured with:
- Robust 6 Sigma Stage-Gate Process
- A dedicated team of 6 Sigma black belts – Key process owners & Quality compliance
- Continuous Improvement & Feedback Loop
Platform
The patented platform offers benefits:
- Web-based end-to-end platform
- Impeccable Quality
- Faster TAT
- Seamless Delivery
People
Dedicated and trained teams:
- 30,000+ collaborators for Data Creation, Labeling & QA
- Credentialed Project Management Team
- Experienced Product Development Team
- Talent Pool Sourcing & Onboarding Team
Process
Highest process efficiency is assured with:
- Robust 6 Sigma Stage-Gate Process
- A dedicated team of 6 Sigma black belts – Key process owners & Quality compliance
- Continuous Improvement & Feedback Loop
Platform
The patented platform offers benefits:
- Web-based end-to-end platform
- Impeccable Quality
- Faster TAT
- Seamless Delivery
Recommended Resources
Buyer’s Guide
Image Annotation & Labeling for Computer Vision
Computer vision is all about making sense of the visual world to train computer vision applications. Its success completely boils down to what we call image annotation – the fundamental process behind the technology that makes machines make intelligent decisions and this is exactly what we are about to discuss and explore.
Blog
How Data Collection Plays a Crucial Role in Developing Facial Recognition Models
Humans are adept at recognizing faces, but we also interpret expressions and emotions quite naturally. Research says we can identify personally familiar faces within 380ms after presentation and 460ms for unfamiliar faces. However, this intrinsically human quality now has a competitor in artificial intelligence and Computer Vision.
Blog
What is AI Image Recognition and How Does it Work?
Human beings have the innate ability to distinguish & precisely identify objects, people, & places from photographs. However, computers don’t come with the capability to classify images. Yet, they can be trained to interpret visual information using computer vision applications & image recognition technology.
Featured Clients
Empowering teams to build world-leading AI products.
Let’s discuss your Training Data needs for Facial Recognition Models
Frequently Asked Questions (FAQ)
Facial recognition is one of the integral components of intelligent biometric security, aimed at confirming or authenticating a person’s identity. As a technology, it is used to ascertain, identify, and categorize humans in videos, photos, and even real-time feeds.
Facial recognition works by matching the captured faces of individuals against a relevant database. The process starts with detection, is followed by a 2D and 3D analysis, image-to-data conversion, and finally matchmaking.
Facial recognition, as an inventive visual identifying technology is often the primal basis for unlocking smartphones and computers. However, its presence in law enforcement i.e. helping officials collect mug shots of the suspects and matching them against databases also qualifies as an example.
If you are planning to train a vertical-specific AI model with computer vision, you must first make it capable of identifying images and faces of individuals and then initiate supervised learning by feeding in newer techniques like semantics, segmentation, and polygon annotation. Facial recognition is therefore the stepping stone for training security-specific AI models, where individual identification is prioritized over object detection.
Facial recognition can be the backbone of several intelligent systems in the post-pandemic era. The benefits include improved retail experience using Face Pay tech, better banking experience, reduced retail crime rates, faster identification of missing persons, improved patient care, accurate attendance tracking, and more.