What is Data Annotation [2025 Updated] – Best Practices, Tools, Benefits, Challenges, Types & more
Need to know the Data Annotation basics? Read this complete Data Annotation guide for beginners to get started.
Curious about how cutting-edge AI systems like self-driving cars or voice assistants achieve their incredible accuracy? The secret lies in high-quality data annotation. This process ensures that data is labeled and categorized precisely, empowering machine learning (ML) models to perform at their best. Whether you’re an AI enthusiast, a business leader, or a tech visionary, this guide will walk you through everything you need to know about data annotation—from the basics to advanced practices.
Why is Data Annotation Critical for AI & ML?
Imagine training a robot to recognize a cat. Without labeled data, the robot sees only pixels—a meaningless jumble. But with data annotation, those pixels are tagged with meaningful labels like “ears,” “tail,” or “fur.” This structured input allows AI to recognize patterns and make predictions.
Key Stat: According to MIT, 80% of data scientists spend more than 60% of their time preparing and annotating data, rather than building models. This highlights how crucial data annotation is as the foundation of AI.
What is Data Annotation?
Data annotation refers to the process of labeling data (text, images, audio, video, or 3D point cloud data) so that machine learning algorithms can process and understand it. For AI systems to work autonomously, they need a wealth of annotated data to learn from.
How It Works in Real-World AI Applications
- Self-Driving Cars: Annotated images and LiDAR data help cars detect pedestrians, roadblocks, and other vehicles.
- Healthcare AI: Labeled X-rays and CT scans teach models to identify abnormalities.
- Voice Assistants: Annotated audio files train speech recognition systems to understand accents, languages, and emotions.
- Retail AI: Product and customer sentiment tagging enables personalized recommendations.
Why Is Data Annotation Essential?
- AI Model Accuracy: The quality of your AI model is only as good as the data it’s trained on. Well-annotated data ensures your models recognize patterns, make accurate predictions, and adapt to new scenarios.
- Diverse Applications: From facial recognition and autonomous driving to sentiment analysis and medical imaging, annotated data powers the most innovative AI solutions across industries.
- Faster AI Development: With the rise of AI-assisted annotation tools, projects can move from concept to deployment at record speed, reducing manual labor and accelerating time-to-market.
The Strategic Importance of Data Annotation for AI Projects
The data annotation landscape continues to evolve rapidly, with significant implications for AI development:
- Market Growth: According to Grand View Research, the global data annotation tools market size is expected to reach $3.4 billion by 2028, growing at a CAGR of 38.5% from 2021 to 2028.
- Efficiency Metrics: Recent studies show AI-assisted annotation can reduce annotation time by up to 70% compared to fully manual methods.
- Quality Impact: IBM research indicates that improving annotation quality by just 5% can increase model accuracy by 15-20% for complex computer vision tasks.
- Cost Factors: Organizations spend an average of $12,000-$15,000 per month on data annotation services for medium-sized projects.
- Adoption Rates: 78% of enterprise AI projects now use a combination of in-house and outsourced annotation services, up from 54% in 2022.
- Emerging Techniques: Active learning and semi-supervised annotation approaches have reduced annotation costs by 35-40% for early adopters.
- Labor Distribution: The annotation workforce has shifted significantly, with 65% of annotation work now performed in specialized annotation hubs in India, Philippines, and Eastern Europe.
Emerging Data Annotation Trends
The data annotation landscape is evolving rapidly, driven by emerging technologies and new industry demands. Here’s what’s making waves this year:
Trend | Description | Impact |
---|---|---|
AI-Assisted Annotation | Smart tools and generative AI models pre-label data, with humans refining results. | Speeds up annotation, reduces costs, and improves scalability. |
Multimodal & Unstructured Data | Annotation now spans text, images, video, audio, and sensor data, often in combination. | Enables richer, more context-aware AI applications. |
Real-Time & Automated Workflows | Automation and real-time annotation are becoming standard, especially for video and streaming data. | Increases efficiency and supports dynamic AI systems. |
Synthetic Data Generation | Generative AI creates synthetic datasets, reducing reliance on manual annotation. | Lowers costs, addresses data scarcity, and boosts model diversity. |
Data Security & Ethics | Stronger focus on privacy, bias mitigation, and compliance with evolving regulations. | Builds trust and ensures responsible AI deployment. |
Specialized Industry Solutions | Custom annotation for healthcare, finance, autonomous vehicles, and more. | Delivers higher accuracy and domain relevance. |
Data Annotation For LLMs?
LLMs, by default, do not understand texts and sentences. They have to be trained to dissect every phrase and word to decipher what a user is exactly looking for and then deliver accordingly. LLM fine-tuning is a crucial step in this process, allowing these models to adapt to specific tasks or domains.
So, when a Generative AI model comes up with the most precision and relevant response to a query – even when presented with the most bizarre questions – it’s accuracy stems from its ability to perfectly comprehend the prompt and its intricacies behind it such as the context, purpose, sarcasm, intent, & more.
Data annotation empowers LLMS with the capabilities to do this. In simple words, data annotation for machine learning involves labeling, categorizing, tagging, and adding any piece of additional attribute to data for machine learning models to process and analyze better. It is only through this critical process that results can be optimized for perfection.
When it comes to annotating data for LLMs, diverse techniques are implemented. While there’s no systematic rule on implementing a technique, it’s generally under the discretion of experts, who analyze the pros and cons of each and deploy the most ideal one.
Let’s look at some of the common data annotation techniques for LLMs.
Manual Annotation: This puts humans in the process of manually annotating and reviewing data. Though this ensures high-quality output, it is tedious and time consuming.
Semi-automatic Annotation: Humans and LLMs work in tandem with each other to tag datasets. This ensures the accuracy of humans and the volume handling capabilities of machines. AI algorithms can analyze raw data and suggest preliminary labels, saving human annotators valuable time. (e.g., AI can identify potential regions of interest in medical images for further human labeling)
Semi-Supervised Learning: Combining a small amount of labeled data with a large amount of unlabeled data to improve model performance.
Automatic Annotation: Time-saving and most ideal to annotate large volumes of datasets, the technique relies on an LLM model’s innate capabilities to tag and add attributes. While it saves time and handles large volumes efficiently, the accuracy depends heavily on the quality and relevance of the pre-trained models.
Instruction Tuning: It refers to fine-tuning language models on tasks described by natural language instructions, involving training on diverse sets of instructions and corresponding outputs.
Zero-shot Learning: Based on existing knowledge and insights, LLMs can deliver labeled data as outputs in this technique. This cuts down expenses in fetching labels and is ideal to process bulk data. This technique involves using a model’s existing knowledge to make predictions on tasks it hasn’t explicitly been trained on.
Prompting: Similar to how a user prompts a model as queries for answers, LLMs can be prompted to annotate data by describing requirements. The output quality here is directly dependent on the prompt quality and how accurate instructions are fed.
Transfer Learning: Using pre-trained models on similar tasks to reduce the amount of labeled data needed.
Active Learning: Here the ML model itself guides the data annotation process. The model identifies data points that would be most beneficial for its learning and requests annotations for those specific points. This targeted approach reduces the overall amount of data that needs to be annotated, leading to Increased efficiency and Improved model performance.
Types of Data Annotation for Modern AI Applications
This is an umbrella term that encompasses different data annotation types. This includes image, text, audio and video. To give you a better understanding, we have broken each down into further fragments. Let’s check them out individually.
Image Annotation
From the datasets they’ve been trained on they can instantly and precisely differentiate your eyes from your nose and your eyebrow from your eyelashes. That’s why the filters you apply fit perfectly regardless of the shape of your face, how close you are to your camera, and more.
So, as you now know, image annotation is vital in modules that involve facial recognition, computer vision, robotic vision, and more. When AI experts train such models, they add captions, identifiers and keywords as attributes to their images. The algorithms then identify and understand from these parameters and learn autonomously.
Image Classification – Image classification involves assigning predefined categories or labels to images based on their content. This type of annotation is used to train AI models to recognize and categorize images automatically.
Object Recognition/Detection – Object recognition, or object detection, is the process of identifying and labeling specific objects within an image. This type of annotation is used to train AI models to locate and recognize objects in real-world images or videos.
Segmentation – Image segmentation involves dividing an image into multiple segments or regions, each corresponding to a specific object or area of interest. This type of annotation is used to train AI models to analyze images at a pixel level, enabling more accurate object recognition and scene understanding.
Image Captioning: Image transcription is the process of pulling details from images and turning them into descriptive text, which is then saved as annotated data. By providing images and specifying what needs to be annotated, the tool produces both the images and their corresponding descriptions.
Optical Character Recognition (OCR): OCR technology allows computers to read and recognize text from scanned images or documents. This process helps accurately extract text and has significantly impacted digitization, automated data entry, and improved accessibility for those with visual impairments.
Pose Estimation (Keypoint Annotation): Pose estimation involves pinpointing and tracking key points on the body, typically at joints, to determine a person’s position and orientation in 2D or 3D space within images or videos.
Audio Annotation
Audio data has even more dynamics attached to it than image data. Several factors are associated with an audio file including but definitely not limited to – language, speaker demographics, dialects, mood, intent, emotion, behavior. For algorithms to be efficient in processing, all these parameters should be identified and tagged by techniques such as timestamping, audio labeling and more. Besides merely verbal cues, non-verbal instances like silence, breaths, even background noise could be annotated for systems to understand comprehensively.
Audio Classification: Audio classification sorts sound data based on its features, allowing machines to recognize and differentiate between various types of audio like music, speech, and nature sounds. It’s often used to classify music genres, which helps platforms like Spotify recommend similar tracks.
Audio Transcription: Audio transcription is the process of turning spoken words from audio files into written text, useful for creating captions for interviews, films, or TV shows. While tools like OpenAI’s Whisper can automate transcription in multiple languages, they may need some manual correction. We provide a tutorial on how to refine these transcriptions using Shaip’s audio annotation tool.
Video Annotation
While an image is still, a video is a compilation of images that create an effect of objects being in motion. Now, every image in this compilation is called a frame. As far as video annotation is concerned, the process involves the addition of keypoints, polygons or bounding boxes to annotate different objects in the field in each frame.
When these frames are stitched together, the movement, behavior, patterns and more could be learnt by the AI models in action. It is only through video annotation that concepts like localization, motion blur and object tracking could be implemented in systems. Various video data annotation software helps you annotate frames. When these annotated frames are stitched together, AI models can learn movement, behavior, patterns, and more. Video annotation is crucial for implementing concepts like localization, motion blur, and object tracking in AI.
Video Classification (Tagging): Video classification involves sorting video content into specific categories, which is crucial for moderating online content and ensuring a safe experience for users.
Video Captioning: Similar to how we caption images, video captioning involves turning video content into descriptive text.
Video Event or Action Detection: This technique identifies and classifies actions in videos, commonly used in sports for analyzing performance or in surveillance to detect rare events.
Video Object Detection and Tracking: Object detection in videos identifies objects and tracks their movement across frames, noting details like location and size as they move through the sequence.
Text Annotation
Today most businesses are reliant on text-based data for unique insight and information. Now, text could be anything ranging from customer feedback on an app to a social media mention. And unlike images and videos that mostly convey intentions that are straight-forward, text comes with a lot of semantics.
As humans, we are tuned to understanding the context of a phrase, the meaning of every word, sentence or phrase, relate them to a certain situation or conversation and then realize the holistic meaning behind a statement. Machines, on the other hand, cannot do this at precise levels. Concepts like sarcasm, humour and other abstract elements are unknown to them and that’s why text data labeling becomes more difficult. That’s why text annotation has some more refined stages such as the following:
Semantic Annotation – objects, products and services are made more relevant by appropriate keyphrase tagging and identification parameters. Chatbots are also made to mimic human conversations this way.
Intent Annotation – the intention of a user and the language used by them are tagged for machines to understand. With this, models can differentiate a request from a command, or recommendation from a booking, and so on.
Sentiment annotation – Sentiment annotation involves labeling textual data with the sentiment it conveys, such as positive, negative, or neutral. This type of annotation is commonly used in sentiment analysis, where AI models are trained to understand and evaluate the emotions expressed in text.
Entity Annotation – where unstructured sentences are tagged to make them more meaningful and bring them to a format that can be understood by machines. To make this happen, two aspects are involved – named entity recognition and entity linking. Named entity recognition is when names of places, people, events, organizations and more are tagged and identified and entity linking is when these tags are linked to sentences, phrases, facts or opinions that follow them. Collectively, these two processes establish the relationship between the texts associated and the statement surrounding it.
Text Categorization – Sentences or paragraphs can be tagged and classified based on overarching topics, trends, subjects, opinions, categories (sports, entertainment and similar) and other parameters.
Lidar Annotation
LiDAR annotation involves labeling and categorizing 3D point cloud data from LiDAR sensors. This essential process helps machines understand spatial information for various uses. For instance, in autonomous vehicles, annotated LiDAR data allows cars to identify objects and navigate safely. In urban planning, it helps create detailed 3D city maps. For environmental monitoring, it aids in analyzing forest structures and tracking changes in terrain. It’s also used in robotics, augmented reality, and construction for accurate measurements and object recognition.
Step-by-Step Data Labeling / Data Annotation Process for Machine Learning Success
The data annotation process involves a series of well-defined steps to ensure high-quality and accurate data labeling process for machine learning applications. These steps cover every aspect of the process, from unstructured data collection to exporting the annotated data for further use. Effective MLOps practices can streamline this process and improve overall efficiency.
Here’s how data annotation team works:
- Data Collection: The first step in the data annotation process is to gather all the relevant data, such as images, videos, audio recordings, or text data, in a centralized location.
- Data Preprocessing: Standardize and enhance the collected data by deskewing images, formatting text, or transcribing video content. Preprocessing ensures the data is ready for annotation task.
- Select the Right Vendor or Tool: Choose an appropriate data annotation tool or vendor based on your project’s requirements.
- Annotation Guidelines: Establish clear guidelines for annotators or annotation tools to ensure consistency and accuracy throughout the process.
- Annotation: Label and tag the data using human annotators or data annotation platform, following the established guidelines.
- Quality Assurance (QA): Review the annotated data to ensure accuracy and consistency. Employ multiple blind annotations, if necessary, to verify the quality of the results.
- Data Export: After completing the data annotation, export the data in the required format. Platforms like Nanonets enable seamless data export to various business software applications.
The entire data annotation process can range from a few days to several weeks, depending on the project’s size, complexity, and available resources.
Advanced Features to Look for in Enterprise Data Annotation Platforms / Data Labeling Tools
Data annotation tools are decisive factors that could make or break your AI project. When it comes to precise outputs and results, the quality of datasets alone doesn’t matter. In fact, the data annotation tools that you use to train your AI modules immensely influence your outputs.
That’s why it is essential to select and use the most functional and appropriate data labeling tool that meets your business or project needs. But what is a data annotation tool in the first place? What purpose does it serve? Are there any types? Well, let’s find out.
Similar to other tools, data annotation tools offer a wide range of features and capabilities. To give you a quick idea of features, here’s a list of some of the most fundamental features you should look for when selecting a data annotation tool.
Dataset Management
The data annotation tool you intend to use must support the high-quality large datasets you have in hand and let you import them into the software for labeling. So, managing your datasets is the primary feature tools offer. Contemporary solutions offer features that let you import high volumes of data seamlessly, simultaneously letting you organize your datasets through actions like sort, filter, clone, merge and more.
Once the input of your datasets is done, next is exporting them as usable files. The tool you use should let you save your datasets in the format you specify so you could feed them into your ML modles. Effective data versioning capabilities are crucial for maintaining dataset integrity throughout the annotation process.
Annotation Techniques
This is what a data annotation tool is built or designed for. A solid tool should offer you a range of annotation techniques for datasets of all types. This is unless you’re developing a custom solution for your needs. Your tool should let you annotate video or images from computer vision, audio or text from NLPs and transcriptions and more. Refining this further, there should be options to use bounding boxes, semantic segmentation, instance segmentation, cuboids, interpolation, sentiment analysis, parts of speech, coreference solution and more.
For the uninitiated, there are AI-powered data annotation tools as well. These come with AI modules that autonomously learn from an annotator’s work patterns and automatically annotate images or text. Such
modules can be used to provide incredible assistance to annotators, optimize annotations and even implement quality checks.
Data Quality Control
Speaking of quality checks, several data annotation tools out there roll out with embedded quality check modules. These allow annotators to collaborate better with their team members and help optimize workflows. With this feature, annotators can mark and track comments or feedback in real time, track identities behind people who make changes to files, restore previous versions, opt for labeling consensus and more.
Security
Since you’re working with data, security should be of highest priority. You may be working on confidential data like those involving personal details or intellectual property. So, your tool must provide airtight security in terms of where the data is stored and how it is shared. It must provide tools that limit access to team members, prevent unauthorized downloads and more.
Apart from these, data security standards and protocols have to be met and complied to.
Workforce Management
A data annotation tool is also a project management platform of sorts, where tasks can be assigned to team members, collaborative work can happen, reviews are possible and more. That’s why your tool should fit into your workflow and process for optimized productivity.
Besides, the tool must also have a minimal learning curve as the process of data annotation by itself is time consuming. It doesn’t serve any purpose spending too much time simply learning the tool. So, it should be intuitive and seamless for anyone to get started quickly.
What are the Benefits of Data Annotation?
Data annotation is crucial to optimizing machine learning systems and delivering improved user experiences. Here are some key benefits of data annotation:
- Improved Training Efficiency: Data labeling helps machine learning models be better trained, enhancing overall efficiency and producing more accurate outcomes.
- Increased Precision: Accurately annotated data ensures that algorithms can adapt and learn effectively, resulting in higher levels of precision in future tasks.
- Reduced Human Intervention: Advanced data annotation tools significantly decrease the need for manual intervention, streamlining processes and reducing associated costs.
Thus, data annotation contributes to more efficient and precise machine learning systems while minimizing the costs and manual effort traditionally required to train AI models.
Quality Control in Data Annotation
Shaip ensures top-notch quality through multiple stages of quality control to ensure quality in data annotation projects.
- Initial Training: Annotators are thoroughly trained on project-specific guidelines.
- Ongoing Monitoring: Regular quality checks during the annotation process.
- Final Review: Comprehensive reviews by senior annotators and automated tools to ensure accuracy and consistency.
Moreover AI can also identify inconsistencies in human annotations and flag them for review, ensuring higher overall data quality. (e.g., AI can detect discrepancies in how different annotators label the same object in an image). So with human and AI the quality of annotation can be improved significantly while reducing the overall time taken to complete the projects.
Overcoming Common Data Annotation Challenges
Data annotation plays a critical role in the development and accuracy of AI and machine learning models. However, the process comes with its own set of challenges:
- Cost of annotating data: Data annotation can be performed manually or automatically. Manual annotation requires significant effort, time, and resources, which can lead to increased costs. Maintaining the quality of the data throughout the process also contributes to these expenses.
- Accuracy of annotation: Human errors during the annotation process can result in poor data quality, directly affecting the performance and predictions of AI/ML models. A study by Gartner highlights that poor data quality costs companies up to 15% of their revenue.
- Scalability: As the volume of data increases, the annotation process can become more complex and time-consuming with larger datasets, especially when working with multimodal data.. Scaling data annotation while maintaining quality and efficiency is challenging for many organizations.
- Data privacy and security: Annotating sensitive data, such as personal information, medical records, or financial data, raises concerns about privacy and security. Ensuring that the annotation process complies with relevant data protection regulations and ethical guidelines is crucial to avoiding legal and reputational risks.
- Managing diverse data types: Handling various data types like text, images, audio, and video can be challenging, especially when they require different annotation techniques and expertise. Coordinating and managing the annotation process across these data types can be complex and resource-intensive.
Organizations can understand and address these challenges to overcome the obstacles associated with data annotation and improve the efficiency and effectiveness of their AI and machine learning projects.
Data Annotation Tool Comparison: Build vs. Buy Decision Framework
One critical and overarching issue that may come up during a data annotation or data labeling project is the choice to either build or buy functionality for these processes. This may come up several times in various project phases, or related to different segments of the program. In choosing whether to build a system internally or rely on vendors, there’s always a trade-off.
As you can likely now tell, data annotation is a complex process. At the same time, it’s also a subjective process. Meaning, there is no one single answer to the question of whether you should buy or build a data annotation tool. A lot of factors need to be considered and you need to ask yourself some questions to understand your requirements and realize if you actually need to buy or build one.
To make this simple, here are some of the factors you should consider.
Your Goal
The first element you need to define is the goal with your artificial intelligence and machine learning concepts.
- Why are you implementing them in your business?
- Do they solve a real-world problem your customers are facing?
- Are they making any front-end or backend process?
- Will you use AI to introduce new features or optimize your existing website, app or a module?
- What is your competitor doing in your segment?
- Do you have enough use cases that need AI intervention?
Answers to these will collate your thoughts – which may currently be all over the place – into one place and give you more clarity.
AI Data Collection / Licensing
AI models require only one element for functioning – data. You need to identify from where you can generate massive volumes of ground-truth data. If your business generates large volumes of data that need to be processed for crucial insights on business, operations, competitor research, market volatility analysis, customer behavior study and more, you need a data annotation tool in place. However, you should also consider the volume of data you generate. As mentioned earlier, an AI model is only as effective as the quality and quantity of data it is fed. So, your decisions should invariably depend on this factor.
If you do not have the right data to train your ML models, vendors can come in quite handy, assisting you with data licensing of the right set of data required to train ML models. In some cases, part of the value that the vendor brings will involve both technical prowess and also access to resources that will promote project success.
Budget
Another fundamental condition that probably influences every single factor we are currently discussing. The solution to the question of whether you should build or buy a data annotation becomes easy when you understand if you have enough budget to spend.
Compliance Complexities
Manpower
Data annotation requires skilled manpower to work on regardless of the size, scale and domain of your business. Even if you’re generating bare minimum data every single day, you need data experts to work on your data for labeling. So, now, you need to realize if you have the required manpower in place.If you do, are they skilled at the required tools and techniques or do they need upskilling? If they need upskilling, do you have the budget to train them in the first place?
Moreover, the best data annotation and data labeling programs take a number of subject matter or domain experts and segment them according to demographics like age, gender and area of expertise – or often in terms of the localized languages they’ll be working with. That’s, again, where we at Shaip talk about getting the right people in the right seats thereby driving the right human-in-the-loop processes that will lead your programmatic efforts to success.
Small and Large Project Operations and Cost Thresholds
In many cases, vendor support can be more of an option for a smaller project, or for smaller project phases. When the costs are controllable, the company can benefit from outsourcing to make data annotation or data labeling projects more efficient.
Companies can also look at important thresholds – where many vendors tie cost to the amount of data consumed or other resource benchmarks. For example, let’s say that a company has signed up with a vendor for doing the tedious data entry required for setting up test sets.
There may be a hidden threshold in the agreement where, for example, the business partner has to take out another block of AWS data storage, or some other service component from Amazon Web Services, or some other third-party vendor. They pass that on to the customer in the form of higher costs, and it puts the price tag out of the customer’s reach.
In these cases, metering the services that you get from vendors helps to keep the project affordable. Having the right scope in place will ensure that project costs do not exceed what is reasonable or feasible for the firm in question.
Open Source and Freeware Alternatives
The do-it-yourself mentality of open source is itself kind of a compromise – engineers and internal people can take advantage of the open-source community, where decentralized user bases offer their own kinds of grassroots support. It won’t be like what you get from a vendor – you won’t get 24/7 easy assistance or answers to questions without doing internal research – but the price tag is lower.
So, the big question – When Should You Buy A Data Annotation Tool:
As with many kinds of high-tech projects, this type of analysis – when to build and when to buy – requires dedicated thought and consideration of how these projects are sourced and managed. The challenges most companies face related to AI/ML projects when considering the “build” option is it’s not just about the building and development portions of the project. There is often an enormous learning curve to even get to the point where true AI/ML development can occur. With new AI/ML teams and initiatives the number of “unknown unknowns” far outweigh the number of “known unknowns.”
Build | Buy |
---|---|
Pros:
| Pros:
|
Cons:
| Cons:
|
To make things even simpler, consider the following aspects:
- when you work on massive volumes of data
- when you work on diverse varieties of data
- when the functionalities associated with your models or solutions could change or evolve in the future
- when you have a vague or generic use case
- when you need a clear idea on the expenses involved in deploying a data annotation tool
- and when you don’t have the right workforce or skilled experts to work on the tools and are looking for a minimal learning curve
If your responses were opposite to these scenarios, you should focus on building your tool.
How to Choose the Right Data Annotation Tool
Selecting the ideal data annotation tool is a critical decision that can make or break your AI project’s success. With a rapidly expanding market and increasingly sophisticated requirements, here’s a practical, up-to-date guide to help you navigate your options and find the best fit for your needs.
A data annotation/labeling tool is a cloud-based or on-premise platform used to annotate high-quality training data for machine learning models. While many rely on external vendors for complex tasks, some use custom-built or open-source tools. These tools handle specific data types like images, videos, text, or audio, offering features like bounding boxes and polygons for efficient labeling.
- Define Your Use Case and Data Types
Start by clearly outlining your project’s requirements:
- What types of data will you be annotating-text, images, video, audio, or a combination?
- Does your use case demand specialized annotation techniques, such as semantic segmentation for images, sentiment analysis for text, or transcription for audio?
Choose a tool that not only supports your current data types but is also flexible enough to accommodate future needs as your projects evolve.
- Evaluate Annotation Capabilities and Techniques
Look for platforms that offer a comprehensive suite of annotation methods relevant to your tasks:
- For computer vision: bounding boxes, polygons, semantic segmentation, cuboids, and keypoint annotation.
- For NLP: entity recognition, sentiment tagging, part-of-speech tagging, and coreference resolution.
- For audio: transcription, speaker diarization, and event tagging.
Advanced tools now often include AI-assisted or automated labeling features, which can speed up annotation and improve consistency.
- Assess Scalability and Automation
Your tool should be able to handle increasing data volumes as your project grows:
- Does the platform offer automated or semi-automated annotation to boost speed and reduce manual effort?
- Can it manage enterprise-scale datasets without performance bottlenecks?
- Are there built-in workflow automation and task assignment features to streamline large team collaborations?
- Prioritize Data Quality Control
High-quality annotations are essential for robust AI models:
- Seek tools with embedded quality control modules, such as real-time review, consensus workflows, and audit trails.
- Look for features that support error tracking, remove duplicate, version control, and easy feedback integration.
- Ensure the platform allows you to set and monitor quality standards from the outset, minimizing error margins and bias.
- Consider Data Security and Compliance
With growing concerns about privacy and data protection, security is non-negotiable:
- The tool should offer robust data access controls, encryption, and compliance with industry standards (like GDPR or HIPAA).
- Evaluate where and how your data is stored-cloud, local, or hybrid options-and whether the tool supports secure sharing and collaboration.
- Decide on Workforce Management
Determine who will annotate your data:
- Does the tool support both in-house and outsourced annotation teams?
- Are there features for task assignment, progress tracking, and collaboration?
- Consider the training resources and support provided for onboarding new annotators.
- Choose the Right Partner, Not Just a Vendor
The relationship with your tool provider matters:
- Look for partners who offer proactive support, flexibility, and a willingness to adapt as your needs change.
- Assess their experience with similar projects, responsiveness to feedback, and commitment to confidentiality and compliance.
Key Takeaway
The best data annotation tool for your project is one that aligns with your specific data types, scales with your growth, guarantees data quality and security, and integrates seamlessly into your workflow. By focusing on these core factors-and choosing a platform that evolves with the latest AI trends-you’ll set your AI initiatives up for long-term success.
Industry-Specific Data Annotation Use Cases and Success Stories
Data annotation is vital in various industries, enabling them to develop more accurate and efficient AI and machine learning models. Here are some industry-specific use cases for data annotation:
Healthcare Data Annotation
Data annotation for medical images is instrumental in developing AI-powered medical image analysis tools. Annotators label medical images (such as X-rays, MRIs) for features like tumors or specific anatomical structures, enabling algorithms to detect diseases and abnormalities with greater accuracy. For example, data annotation is crucial for training machine learning models to identify cancerous lesions in skin cancer detection systems. Additionally, data annotators label electronic medical records (EMRs) and clinical notes, aiding in the development of computer vision systems for disease diagnosis and automated medical data analysis.
Retail Data Annotation
Retail data annotation involves labeling product images, customer data, and sentiment data. This type of annotation helps create and train AI/ML models to understand customer sentiment, recommend products, and enhance the overall customer experience.
Finance Data Annotation
The financial sector utilizes data annotation for fraud detection and sentiment analysis of financial news articles. Annotators label transactions or news articles as fraudulent or legitimate, training AI models to automatically flag suspicious activity and identify potential market trends. For instance, high-quality annotations help financial institutions train AI models to recognize patterns in financial transactions and detect fraudulent activities. Moreover, financial data annotation focuses on annotating financial documents and transactional data, essential for developing AI/ML systems that detect fraud, address compliance issues, and streamline other financial processes.
Automotive Data Annotation
Data annotation in the automotive industry involves labeling data from autonomous vehicles, such as camera and LiDAR sensor information. This annotation helps create models to detect objects in the environment and process other critical data points for autonomous vehicle systems.
Industrial or Manufaturing Data Annotation
Data annotation for manufacturing automation fuels the development of intelligent robots and automated systems in manufacturing. Annotators label images or sensor data to train AI models for tasks like object detection (robots picking items from a warehouse) or anomaly detection (identifying potential equipment malfunctions based on sensor readings). For example, data annotation enables robots to recognize and grasp specific objects on a production line, improving efficiency and automation. Additionally, industrial data annotation is used to annotate data from various industrial applications, including manufacturing images, maintenance data, safety data, and quality control information. This type of data annotation helps create models capable of detecting anomalies in production processes and ensuring worker safety.
E-commerce Data Annotation
Annotating product images and user reviews for personalized recommendations and sentiment analysis.
What are the best practices for data annotation?
To ensure the success of your AI and machine learning projects, it’s essential to follow best practices for data annotation. These practices can help enhance the accuracy and consistency of your annotated data:
- Choose the appropriate data structure: Create data labels that are specific enough to be useful but general enough to capture all possible variations in data sets.
- Provide clear instructions: Develop detailed, easy-to-understand data annotation guidelines and best practices to ensure data consistency and accuracy across different annotators.
- Optimize the annotation workload: Since annotation can be costly, consider more affordable alternatives, such as working with data collection services that offer pre-labeled datasets.
- Collect more data when necessary: To prevent the quality of machine learning models from suffering, collaborate with data collection companies to gather more data if required.
- Outsource or crowdsource: When data annotation requirements become too large and time-consuming for internal resources, consider outsourcing or crowdsourcing.
- Combine human and machine efforts: Use a human-in-the-loop approach with data annotation software to help human annotators focus on the most challenging cases and increase the diversity of the training data set.
- Prioritize quality: Regularly test your data annotations for quality assurance purposes. Encourage multiple annotators to review each other’s work for accuracy and consistency in labeling datasets.
- Ensure compliance: When annotating sensitive data sets, such as images containing people or health records, consider privacy and ethical issues carefully. Non-compliance with local rules can damage your company’s reputation.
Adhering to these data annotation best practices can help you guarantee that your data sets are accurately labeled, accessible to data scientists, and ready to fuel your data-driven projects.
Case Studies / Success Stories
Here are some specific case study examples that address how data annotation and data labeling really work on the ground. At Shaip, we take care to provide the highest levels of quality and superior results in data annotation and data labeling. Much of the above discussion of standard achievements for effective data annotation and data labeling reveals how we approach each project, and what we offer to the companies and stakeholders we work with.
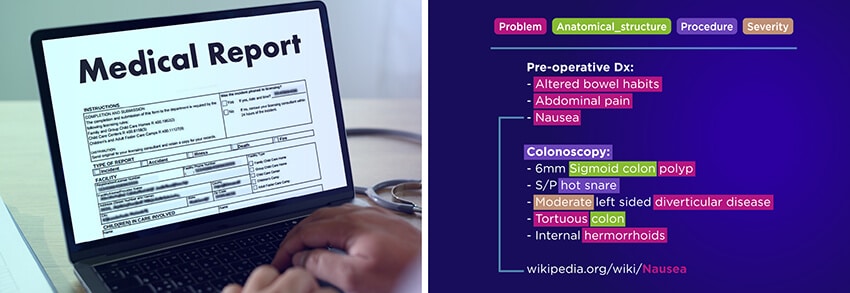
In one of our recent clinical data licensing projects, we processed over 6,000 hours of audio, carefully removing all protected health information (PHI) to ensure the content met HIPAA standards. After de-identifying the data, it was ready to be used for training healthcare speech recognition models.
In projects like these, the real challenge lies in meeting the strict criteria and hitting key milestones. We start with raw audio data, which means there’s a big focus on de-identifying all the parties involved. For example, when we use Named Entity Recognition (NER) analysis, our goal isn’t just to anonymize the information, but also to make sure it’s properly annotated for the models.
Another case study that stands out is a massive conversational AI training data project where we worked with 3,000 linguists over 14 weeks. The result? We produced AI model training data in 27 different languages, helping develop multilingual digital assistants that can engage with people in their native languages.
This project really underscored the importance of getting the right people in place. With such a large team of subject matter experts and data handlers, keeping everything organized and streamlined was crucial to meet our deadline. Thanks to our approach, we were able to complete the project well ahead of the industry standard.
In another example, one of our healthcare clients needed top-tier annotated medical images for a new AI diagnostic tool. By leveraging Shaip’s deep annotation expertise, the client improved their model’s accuracy by 25%, resulting in quicker and more reliable diagnoses.
We’ve also done a lot of work in areas like bot training and text annotation for machine learning. Even when working with text, privacy laws still apply, so de-identifying sensitive information and sorting through raw data is just as important.
Across all these different data types—whether it’s audio, text, or images—our team at Shaip has consistently delivered by applying the same proven methods and principles to ensure success, every time.
Wrapping Up
Key Takeaways
- Data annotation is the process of labeling data to train machine learning models effectively
- High-quality data annotation directly impacts AI model accuracy and performance
- The global data annotation market is projected to reach $3.4 billion by 2028, growing at 38.5% CAGR
- Choosing the right annotation tools and techniques can reduce project costs by up to 40%
- Implementation of AI-assisted annotation can improve efficiency by 60-70% for most projects
We honestly believe this guide was resourceful to you and that you have most of your questions answered. However, if you’re still not convinced about a reliable vendor, look no further.
We, at Shaip, are a premier data annotation company. We have experts in the field who understand data and its allied concerns like no other. We could be your ideal partners as we bring to table competencies like commitment, confidentiality, flexibility and ownership to each project or collaboration.
So, regardless of the type of data you intend to get accurate annotations for, you could find that veteran team in us to meet your demands and goals. Get your AI models optimized for learning with us.
Transform Your AI Projects with Expert Data Annotation Services
Ready to elevate your machine learning and AI initiatives with high-quality annotated data? Shaip offers end-to-end data annotation solutions tailored to your specific industry and use case.
Why Partner with Shaip for Your Data Annotation Needs:
- Domain Expertise: Specialized annotators with industry-specific knowledge
- Scalable Workflows: Handle projects of any size with consistent quality
- Customized Solutions: Tailored annotation processes for your unique needs
- Security & Compliance: HIPAA, GDPR, and ISO 27001 compliant processes
- Flexible Engagement: Scale up or down based on project requirements
Let’s Talk
Frequently Asked Questions (FAQ)
1. What is data annotation or Data labeling?
Data Annotation or Data Labeling is the process that makes data with specific objects recognizable by machines so as to predict the outcome. Tagging, transcribing or processing objects within textual, image, scans, etc. enable algorithms to interpret the labeled data and get trained to solve real business cases on its own without human intervention.
2. What is annotated data?
In machine learning (both supervised or unsupervised), labeled or annotated data is tagging, transcribing or processing the features you want your machine learning models to understand and recognize so as to solve real world challenges.
3. Who is a Data Annotator?
A data annotator is a person who works tirelessly to enrich the data so as to make it recognizable by machines. It may involve one or all of the following steps (subject to the use case in hand and the requirement): Data Cleaning, Data Transcribing, Data Labeling or Data Annotation, QA etc.
4. Why is data annotation important for AI and ML?
AI models require labeled data to recognize patterns and perform tasks like classification, detection, or prediction. Data annotation ensures that models are trained on high-quality, structured data, leading to better accuracy, performance, and reliability.
5. How do I ensure the quality of annotated data?
- Provide clear annotation guidelines to your team or vendor.
- Use quality assurance (QA) processes, such as blind reviews or consensus models.
- Leverage AI tools to flag inconsistencies and errors.
- Perform regular audits and sampling to ensure data accuracy.
6. What is the difference between manual and automated annotation?
Manual Annotation: Done by human annotators, ensuring high accuracy but requiring significant time and cost.
Automated Annotation: Uses AI models for labeling, offering speed and scalability. However, it may require human review for complex tasks.
A semi-automatic approach (human-in-the-loop) combines both methods for efficiency and precision.
7. What are pre-labeled datasets, and should I use them?
Pre-labeled datasets are ready-made datasets with annotations, often available for common use cases. They can save time and effort but may need customization to fit specific project requirements.
8. How does data annotation differ for supervised, unsupervised, and semi-supervised learning?
In supervised learning, labeled data is crucial for training models. Unsupervised learning typically does not require annotation, while semi-supervised learning uses a mix of labeled and unlabeled data.
9. How is generative AI impacting data annotation?
Generative AI is increasingly used to pre-label data, while human experts refine and validate annotations, making the process faster and more cost-efficient.
10. What ethical and privacy concerns should be considered?
Annotating sensitive data requires strict compliance with privacy regulations, robust data security, and measures to minimize bias in labeled datasets.
11. How should I budget for data annotation?
Budget depends on how much data you need labeled, the complexity of the task, the type of data (text, image, video), and whether you use in-house or outsourced teams. Using AI tools can reduce costs. Expect prices to vary widely based on these factors.
12. What hidden costs should I watch out for?
Costs can include data security, fixing annotation errors, training annotators, and managing large projects.
13. How much annotated data do I need?
It depends on your project’s goals and model complexity. Start with a small labeled set, train your model, then add more data as needed to improve accuracy. More complex tasks usually need more data.